The Dual Nature of AI Privacy in Society
Artificial Intelligence (AI) has transformed many aspects of our lives. It automates mundane tasks and revolutionizes industries like healthcare, finance, and communication. These innovations enable remarkable progress but also introduce significant privacy challenges. As AI becomes more integrated into our lives, balancing its capabilities with the right to privacy becomes more difficult. This blog explores AI privacy, data protection challenges, potential solutions, and the broader impact on society.
Understanding AI Privacy and Data Dependency
AI systems, especially those using machine learning (ML), need large amounts of data to perform well. Their impressive capabilities come from this data. From voice recognition to recommendation algorithms, AI excels at understanding the world. It relies on analyzing and learning from the data it collects.
The more data these systems access, the better they become at making predictions. They recognize patterns and deliver personalized experiences. This reliance on data, which often includes personal or sensitive information, fuels AI’s growth. Data serves as the lifeblood of AI, enabling continuous improvement. However, this relationship creates a delicate balance between innovation and privacy. It poses significant ethical and regulatory challenges.
Why AI Needs Data
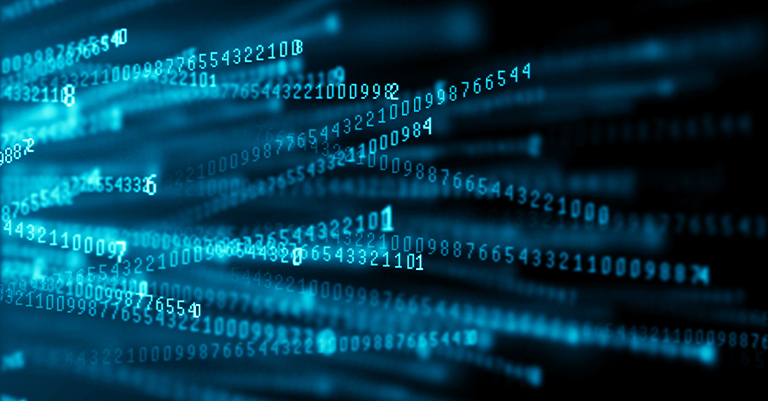
AI algorithms, especially those in deep learning (DL) and natural language processing (NLP), need large datasets to develop their models. These algorithms mimic human thought processes by analyzing data, learning from it, and improving their accuracy. For example, an image recognition model may need thousands or millions of labeled images. This helps it learn to differentiate between objects like cats and dogs. Similarly, NLP models use large text datasets to understand language nuances, including context, tone, and intent.
Training and Generalization
During training, AI models are given data to recognize patterns and relationships. Larger and more diverse datasets help the model generalize better. This improves its accuracy when predicting new, unseen data. As a result, AI can progress from basic tasks like identifying objects to more complex ones. These include interpreting medical images or predicting financial market trends.
Fine-Tuning Models
After the initial training, AI systems often undergo fine-tuning with new data. This helps them adapt to changing conditions or preferences. For example, recommendation algorithms on platforms like Netflix or Spotify update continuously based on user interactions. They refine their suggestions as they learn more about user preferences over time.
Personalization
AI’s ability to offer personalized experiences is one of its strongest features. However, it requires a deep understanding of individual users. Personalization means tailoring recommendations, ads, or interactions based on data like browsing history and purchase behavior. It can also involve location and time spent engaging with specific content. This explains why streaming services often know what show you might like next. It also clarifies why online ads match recent searches so closely.
The success of these AI applications relies on access to rich, diverse datasets. Without this constant flow of data, AI systems would struggle. They would find it hard to maintain the precision and personalization that users expect.
Types of Data Involved
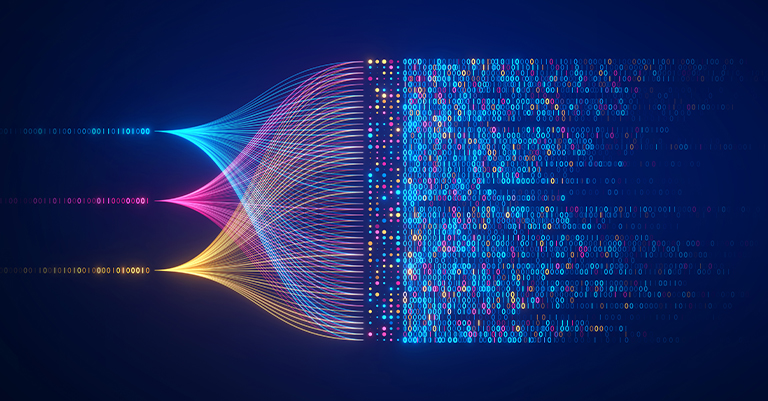
The range of data that AI systems use varies widely, covering both non-personal information and highly sensitive personal data. The type of data collected can influence the degree of privacy concerns, as well as the regulatory implications involved. Here are the key types of data used by AI:
Non-Personal Data
This includes information not linked to a specific person, like weather patterns, traffic data, or general market trends. While non-personal data might seem less invasive, it can still provide valuable insights when combined with other datasets. For example, traffic and weather data can help predict the best routes for ride-sharing services, improving user experiences.
Personal Data
This category includes any information that can be directly or indirectly linked to a person. Examples are names, addresses, emails, and IP addresses. Personal data is essential for services like targeted advertising, social media, and e-commerce websites. It helps these platforms understand and meet individual user needs.
Sensitive Data
Sensitive personal data includes health records, financial information, biometric data, and social media activity. This data needs stronger protection because it can be misused. AI systems in healthcare, for example, use sensitive patient data to improve diagnostics and treatment recommendations. However, breaches in this area could seriously impact individuals’ privacy and security.
Behavioral Data
This includes information about how users interact with digital platforms, such as browsing history, clicks, time spent on pages, and even cursor movements. Behavioral data is especially valuable for companies seeking to optimize user experience or boost engagement on their platforms. For instance, e-commerce sites use behavioral data to personalize product suggestions and improve the shopping experience.
Proprietary Data
This refers to information that is specific to an organization or individual and often gets classified as confidential. Examples include internal business reports, client lists, and proprietary algorithms. For companies that leverage AI to gain a competitive edge, protecting proprietary data is essential for maintaining their market position.
Each of these data types is important for AI systems’ functioning. However, their use raises privacy concerns. When AI systems access and process various data types, people worry about how much they know about us. They also worry about how these systems use or share that information.
The Privacy-Utility Trade-Off
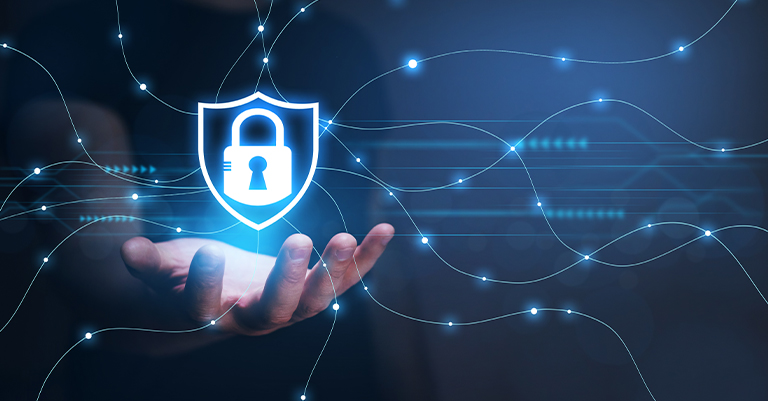
The inherent reliance on data drives the primary privacy concerns associated with AI. On one hand, AI’s need for extensive data sets allows it to perform complex functions like recognizing diseases from medical images or predicting user needs before they even express them. On the other hand, this dependency raises questions about where to draw the line between utility and intrusion.
Improved Functionality vs. Privacy Concerns
The more data an AI system has, the better it performs. For example, a smart home assistant can give more personalized responses if it knows a household’s schedule, routines, and preferences. However, this raises concerns that the assistant might be “listening” constantly or collecting more information than needed, potentially invading users’ privacy.
Transparency Challenges
It’s hard for users to understand how AI systems use data. Many AI systems act like “black boxes,” making decisions based on data in unclear ways. This lack of transparency makes it difficult for users to make informed choices about their data. Users are often left uncertain about how much information they are sharing.
Data Retention
After collecting data, questions arise about how long organizations store it and why. AI systems might store user data to improve performance, but long-term storage increases the risk of breaches or misuse. For example, storing data indefinitely for personalization can conflict with users’ privacy expectations and their right to delete data. As AI systems become more advanced, establishing clear boundaries and ethical guidelines around data use is essential. It’s crucial to balance AI’s benefits with privacy protection, allowing users to enjoy advancements without feeling that their boundaries have been violated.
Key Concerns Regarding AI Privacy
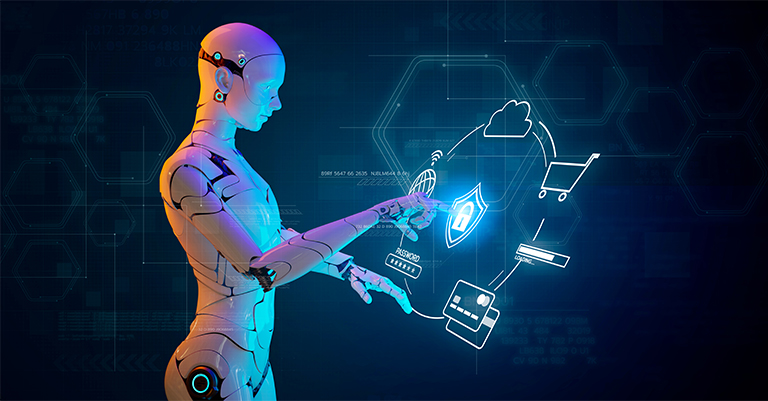
The rapid advancement of AI technologies has opened up new avenues for efficiency, automation, and personalized user experiences. However, this evolution has also brought about a range of privacy issues that can affect both individuals and organizations. As AI systems become more integrated into our daily lives, understanding these key concerns is critical to navigating the complex relationship between technological innovation and the right to privacy. Below, we delve deeper into the primary privacy concerns surrounding AI.
Data Collection Without Consent
A major issue with AI is how much data it collects and processes, often without users’ explicit consent. Many apps and services use AI to analyze data collected through background tracking, cookies, and device sensors. Users may not fully understand or even know about these mechanisms. This data collection often lacks transparency, leaving users unaware of how much data is gathered.
Lack of Transparency
Many users accept terms and conditions without fully understanding them, allowing companies to collect large amounts of data through AI. For example, mobile apps often request access to a user’s location, microphone, or contact list, even when it’s not essential for the app’s function.
Impact on Users
Extensive data collection can make users feel their privacy is violated, leading to mistrust of AI-powered services. It limits their control over shared information, as opting out often means losing access to the service. This lack of clear consent options reduces user trust and exposes companies to regulatory risks. This is especially true in regions with strict data laws, like the GDPR, which require clear and informed user consent.
Data Security Risks
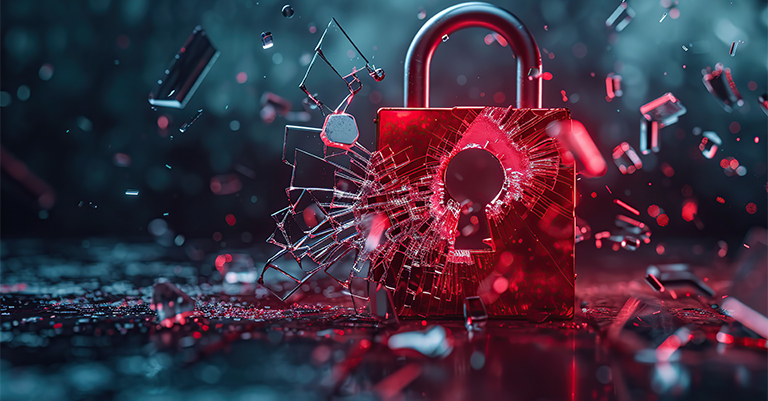
Given their reliance on large datasets, cybercriminals often target AI systems, making data security a significant concern. AI models frequently handle sensitive information, including personal addresses, identification numbers, financial records, and medical histories. If hackers breach an AI system, the potential for harm can be extensive.
Data Breaches
The healthcare industry provides a clear example of the risks involved. AI systems increasingly manage patient records, diagnose conditions, and optimize treatment plans. However, breaches in these systems can expose sensitive medical information, potentially leading to identity theft or other forms of exploitation. The consequences affect not just individuals; organizations also suffer reputational damage, legal repercussions, and financial losses.
Concerns with Cloud Storage
Many AI systems depend on cloud-based storage solutions to process and analyze data efficiently. While the cloud offers scalability and easy access, it also introduces vulnerabilities. If data stored in the cloud is not secured properly, it can be at risk. Without encryption or access controls, it becomes susceptible to unauthorized access or exposure during a breach.
AI Models as Attack Vectors
Beyond breaching databases, attackers can manipulate AI models directly. Adversarial attacks can alter input data to trick AI systems into making wrong decisions. This compromises data security and can lead to flawed outcomes, like incorrect medical diagnoses or inaccurate facial recognition in security systems.
Bias and Discrimination
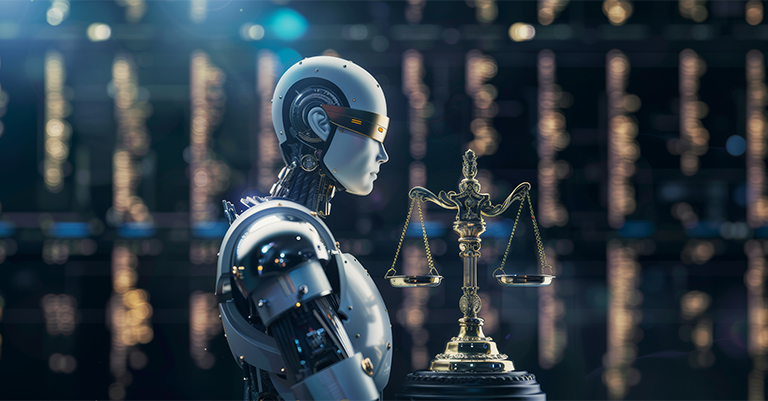
AI models are only as unbiased as the data they are trained on. When training data includes social biases—like race, gender, age, or socioeconomic status—these biases can influence the AI’s decisions. This can create unfair outcomes that harm certain groups. For example, AI recruitment tools have sometimes favored specific demographics because of biases in past hiring data. If a company’s past hiring favored male candidates, an AI trained on that data might continue those biases, disadvantaging female applicants.
Bias Amplification
AI systems can inadvertently amplify biases. In social media algorithms, for example, AI may promote content that aligns with the preferences of a majority group. This practice can sideline diverse perspectives. It can also create echo chambers and contribute to polarization in online communities.
Privacy Concern
Biased AI systems can feel like a violation of personal rights, especially when they impact access to opportunities, services, or social status. When AI-driven assumptions lead to profiling, users may face unequal treatment, raising serious ethical and privacy concerns. To address this, AI developers should use fairness audits, bias detection tools, and diverse training datasets. However, achieving complete fairness in AI is challenging, as even the best efforts can struggle to remove deeply ingrained biases.
Surveillance and Invasion of Privacy
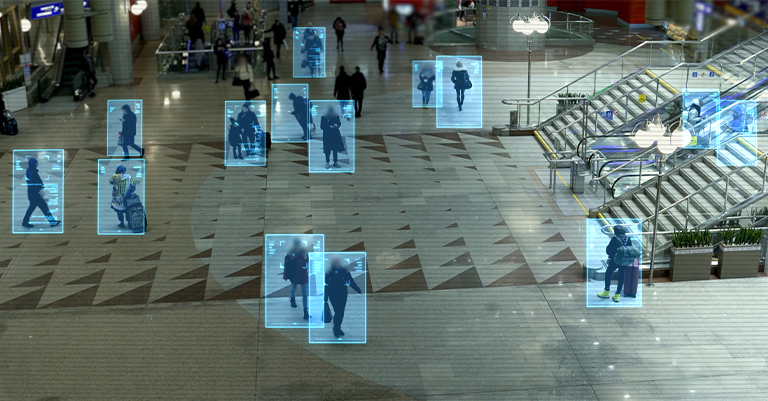
AI-powered surveillance technologies, like facial recognition and behavior analytics, are becoming more common, raising concerns about their ethical use. Governments, law enforcement, and private companies can use these tools for monitoring people in ways previously impossible. Often, this monitoring happens without individuals’ knowledge or consent, leading to serious privacy concerns.
Facial Recognition Technology
This technology is now widely used in public spaces, from airports and train stations to retail stores. While facial recognition can enhance security by identifying suspects or missing persons, it can also be used to track ordinary citizens, raising fears of mass surveillance.
Behavioral Analytics
AI systems that analyze behaviors—such as gait recognition or even emotional analysis through facial expressions—are becoming increasingly sophisticated. While these technologies can be useful for detecting threats, their use in everyday settings can feel like a deep invasion of personal space.
Implications
The widespread use of surveillance AI can undermine the sense of anonymity people expect in public spaces. This creates a society where individuals feel constantly watched, leading to self-censorship and reduced personal freedoms. People may become more conscious of their behavior in areas under surveillance.
The deployment of AI surveillance tools raises critical questions about the balance between public safety and individual privacy. Without clear regulations and transparency, there is a risk that these technologies will be misused, leading to a loss of public trust and potentially undermining democratic values.
Data Retention and Erasure Issues
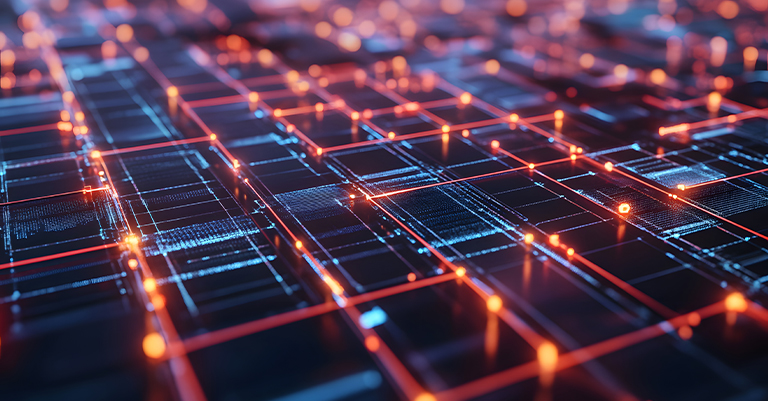
Another major concern with AI is the tendency to store data for extended periods, sometimes even indefinitely. AI systems often need to retain historical data to continue learning and adapting, but this can make it difficult for users to control their digital footprint or exercise their right to privacy.
Right to Be Forgotten
With growing awareness of privacy rights, users increasingly demand the right to have their data deleted or forgotten. This is especially relevant in regions governed by laws like the GDPR. These laws give individuals the right to request the erasure of their personal data. However, implementing these rights in AI systems is challenging. AI systems often learn from and adapt based on the data they collect.
Persistent Data in AI Models
Even if a user’s data is deleted from a database, an AI model might retain knowledge derived from that data. For example, a facial recognition system trained with a user’s images may still retain aspects of that training after the images are deleted. This raises concerns about whether true data erasure is possible in AI systems.
Long-Term Data Storage Risks
The longer data is stored, the more vulnerable it becomes to unauthorized access, especially if security measures are outdated. This increases the risk of data breaches over time, potentially exposing sensitive information long after it was collected.
These issues show the need for AI systems to use better data management practices, like time-based retention and reducing reliance on individual data points. They also stress the importance of building AI with privacy in mind initially, rather than adding it later.
The Legal Landscape and Regulatory Frameworks
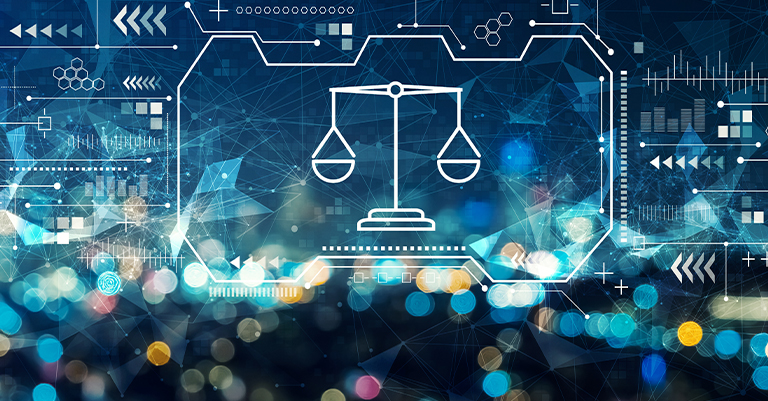
As the influence of artificial intelligence (AI) continues to expand, concerns about privacy and data security have become more prominent, prompting the development of various regulatory frameworks and guidelines. These regulations aim to strike a balance between protecting users’ privacy and allowing technological innovation to thrive. While some regulations are binding and enforce strict data protection standards, others offer guidelines to encourage ethical practices in AI development. Here, we explore the most significant regulations and frameworks and their impact on AI.
General Data Protection Regulation (GDPR) – Europe
The General Data Protection Regulation (GDPR), implemented in the European Union in 2018, has set a global benchmark for data privacy. Its provisions prioritize user consent, data minimization, and transparency, providing individuals with robust control over their personal data. As a result, it has become a reference point for data privacy laws worldwide, influencing regulations beyond Europe.
Key Provisions
The GDPR requires organizations to obtain explicit, informed consent before they collect personal data from users. This ensures that individuals understand how their data will be used. They also have the right to refuse or withdraw consent at any time. The regulation allows users to access their data, correct inaccuracies, and request deletion under the “right to be forgotten.” Organizations must apply privacy-by-design principles, meaning they should consider privacy at every stage of data processing.
Impact on AI
Global Influence
The GDPR’s influence extends beyond the EU, as many global companies choose to adopt GDPR standards in regions outside of Europe to simplify compliance across different markets. This has raised the global standard for data privacy, prompting other countries to consider similar regulations.
California Consumer Privacy Act (CCPA) – United States
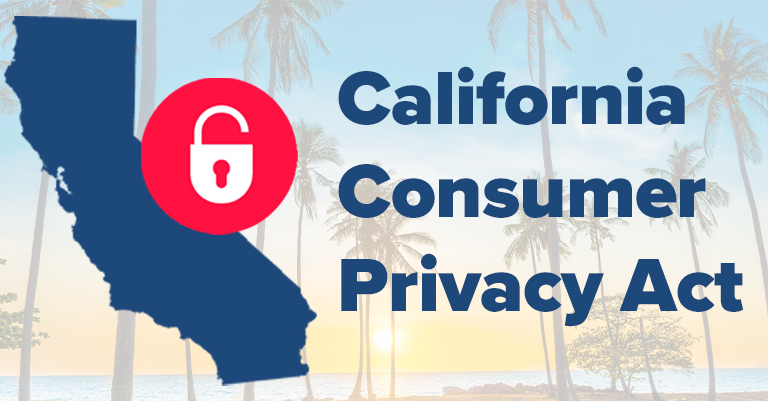
The California Consumer Privacy Act (CCPA), which came into effect in 2020, is one of the most comprehensive privacy laws in the United States. It aims to empower consumers with greater control over their personal data, setting a new standard for data privacy in the U.S. and serving as a catalyst for similar legislative efforts across other states.
Consumer Rights
The CCPA grants California residents the right to know what personal information businesses collect, the purpose for which it is collected, and whether it will be sold to third parties. It also allows consumers to request that businesses delete their personal data and opt out of the sale of their data. Importantly, the CCPA introduces the concept of data portability, requiring companies to provide users with their data in a readily usable format.
Implications for AI
Compliance with the CCPA can limit how AI systems use personal data for training and refining algorithms. For instance, companies must ensure that their data collection practices align with users’ rights to access and delete their data. This can complicate the training of AI models, as companies must be prepared to remove a user’s data from their training datasets if requested. The CCPA also introduces a financial component, allowing consumers to seek damages if their data is misused or if a data breach occurs due to negligence, which adds a financial incentive for businesses to strengthen their data privacy practices.
A Model for Other States
The CCPA has inspired similar legislative proposals in other U.S. states, such as the Virginia Consumer Data Protection Act (VCDPA) and the Colorado Privacy Act (CPA). As more states adopt similar laws, the patchwork of state regulations may push the U.S. towards a more unified federal privacy standard in the future.
AI Ethics Guidelines and Frameworks
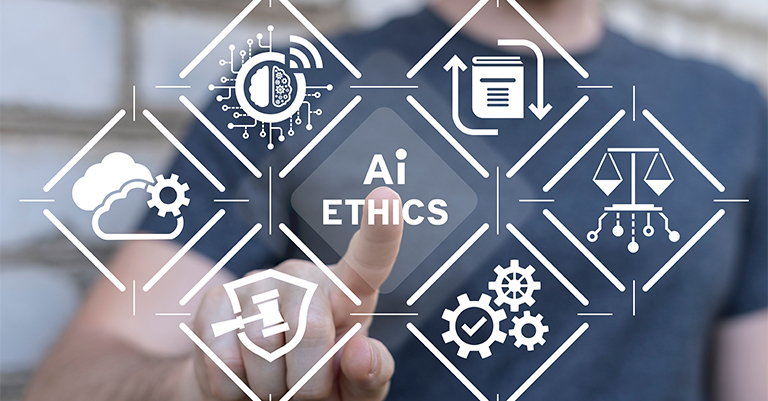
In addition to legally binding regulations, several countries, organizations, and international bodies have developed ethical guidelines for AI. These frameworks focus on principles like fairness, accountability, transparency, and human rights, aiming to guide the responsible development and deployment of AI technologies.
The Organization for Economic Co-operation and Development (OECD) has established a set of AI principles that promote human-centric AI development. These principles emphasize the importance of accountability, transparency, and explainability in AI systems, encouraging organizations to prioritize ethical considerations alongside technological progress. Similarly, the European Union has published its “Ethics Guidelines for Trustworthy AI,” which outlines seven key requirements for AI, including transparency, diversity, non-discrimination, and societal well-being.
Industry-Led Initiatives
In addition to governmental guidelines, tech companies and industry groups have also created ethical AI frameworks. For example, the Partnership on AI, which includes members like Google, Microsoft, and IBM, has developed principles to guide the ethical deployment of AI in areas such as fairness, transparency, and AI safety. These industry-led initiatives often focus on voluntary best practices and encourage self-regulation.
Challenges
One significant challenge with ethical guidelines is their non-binding nature. Unlike laws such as the GDPR or the CCPA, these guidelines lack legal enforcement, meaning organizations are not legally required to follow them. As a result, adherence to these ethical principles varies widely among companies, with some embracing them fully and others using them as marketing tools without substantive changes in practice. Additionally, the abstract nature of many ethical principles can make it difficult to apply them consistently in real-world AI applications.
Emerging Global Regulations and Trends
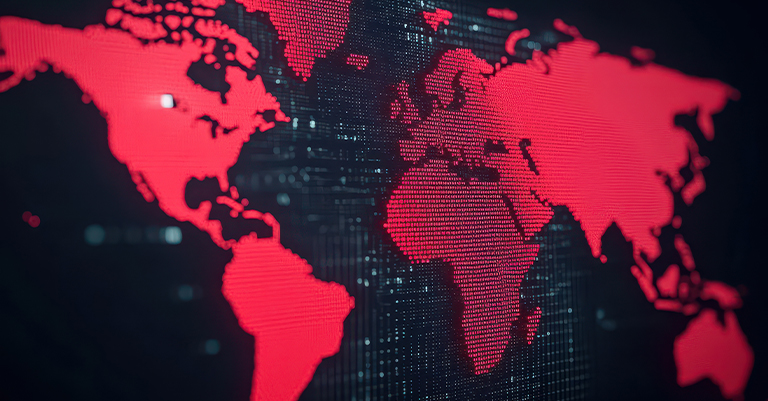
While the GDPR and CCPA represent landmark legislation in data privacy, many countries are now developing their own regulations to address the unique challenges posed by AI. This evolving global landscape reflects a growing recognition of the need to balance innovation with the protection of personal rights.
China’s Personal Information Protection Law (PIPL)
China has introduced the Personal Information Protection Law (PIPL), which mirrors several aspects of the GDPR, such as user consent and data security requirements. However, the PIPL also reflects China’s approach to balancing data privacy with state surveillance needs, allowing the government to access data under certain conditions. The PIPL introduces strict penalties for non-compliance, signaling China’s intent to improve data privacy standards within its borders.
Canada’s Bill C-27
Canada has proposed Bill C-27, which includes the Consumer Privacy Protection Act (CPPA) and the Artificial Intelligence and Data Act (AIDA). The CPPA aims to enhance individual control over personal data, while AIDA seeks to regulate AI systems that may pose risks of bias, discrimination, or harm. This dual focus on privacy and AI-specific risks highlights the emerging trend of countries crafting regulations that address both data protection and AI accountability.
The Future of AI Regulation in the United States
The U.S. has yet to enact a comprehensive federal privacy law, but there is increasing pressure to develop one, especially as states continue to adopt their own regulations. Proposed federal legislation, such as the American Data Privacy Protection Act (ADPPA), aims to create a nationwide standard for data privacy that would preempt state laws, simplifying compliance for companies operating across multiple states.
Global Convergence vs. Divergence
While many countries are aligning their data privacy laws with global standards like the GDPR, there is also a divergence in regulatory approaches, particularly between Western democracies and countries with different governance models. This creates a complex environment for multinational companies, which must navigate varying compliance requirements across regions while maintaining consistent AI practices.
The Role of AI-Specific Legislation
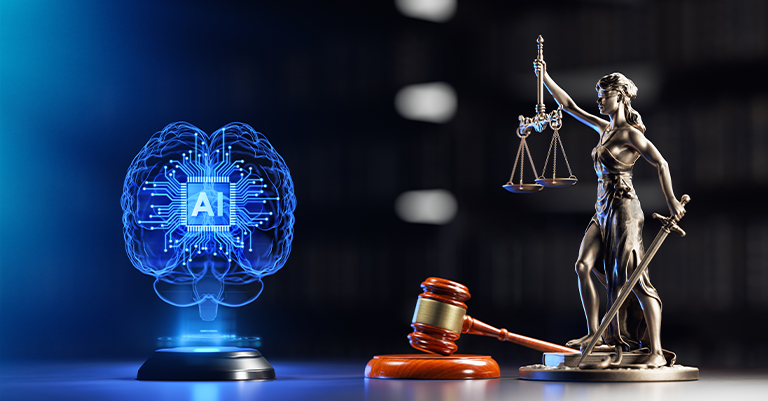
As AI technologies evolve, there is a growing recognition that existing data privacy laws may not fully address the unique challenges posed by AI. Some jurisdictions are now developing regulations that focus specifically on AI, addressing issues such as algorithmic accountability, bias prevention, and transparency.
European Union’s AI Act
The European Union has proposed the AI Act, which aims to create a legal framework for AI development and use. It categorizes AI applications into risk levels—unacceptable risk, high risk, and low risk—based on their potential impact on individuals’ rights and safety. High-risk AI applications, such as those used in healthcare or law enforcement, would be subject to stricter oversight, including requirements for transparency, risk assessment, and human oversight.
AI Audits and Certifications
The concept of AI audits is gaining traction as a way to ensure that AI systems adhere to ethical and regulatory standards. AI audits can involve assessing an AI system’s compliance with transparency requirements, evaluating its impact on users, and verifying that it meets fairness and non-discrimination criteria. Some experts advocate for creating certification systems, similar to ISO standards, that would verify the ethical and privacy compliance of AI systems.
Addressing the Black Box Problem
Many AI-specific regulations focus on increasing the transparency of AI systems, particularly those that make critical decisions affecting individuals’ lives. The goal is to make AI decision-making more understandable and to provide users with the ability to challenge decisions made by AI systems. This focus on transparency is a direct response to concerns that users may not fully understand how AI influences their lives.
Balancing Innovation and Privacy: The Path Forward
The evolving regulatory landscape for AI and privacy represents a global effort to balance the benefits of AI with the need to protect individuals’ rights. While laws like the GDPR and CCPA provide a foundation for data protection, emerging AI-specific regulations and ethical guidelines reflect the growing recognition that AI poses unique challenges that require tailored solutions. Moving forward, a collaborative approach that involves governments, industry leaders, and civil society will be essential to ensure that AI development aligns with privacy and human rights principles. This path forward must prioritize both innovation and the public’s trust, ensuring that the benefits of AI are realized without compromising individual freedoms and privacy.
Potential Solutions and Best Practices for AI Privacy
The privacy challenges associated with AI can be complex, but several effective strategies and best practices can help address these issues. These solutions aim to protect user data while maintaining the functionality and innovation that AI offers. By adopting these methods, organizations can better align their AI systems with privacy standards, building trust with users and complying with regulatory requirements. Here are some key strategies:
Privacy by Design
Privacy by design is a proactive approach that integrates privacy considerations into every stage of the AI development process, from the initial design to deployment and beyond. Instead of treating privacy as an afterthought, this approach ensures that data protection is a core component of the system’s architecture, helping to prevent privacy issues before they arise.
Data Minimization
This principle involves collecting only the data that is absolutely necessary for the AI system to perform its intended function. By limiting the scope of data collection, organizations can reduce the potential risks associated with data storage and breaches. Data minimization also aligns with regulatory requirements like the GDPR, which emphasizes the importance of collecting only relevant and necessary data. For example, a recommendation engine for a music streaming service might only need information about users’ listening history rather than their entire browsing history.
Anonymization and Encryption
Anonymization involves altering data so that individuals cannot be identified directly from the dataset. Encryption, on the other hand, ensures that even if data is intercepted during transmission or storage, it cannot be accessed without the proper decryption key. These techniques are essential for protecting sensitive information, especially when data is stored in cloud environments or shared across different AI systems. Anonymized data can still be used for training AI models while significantly reducing the privacy risks associated with handling personal information.
Differential Privacy
A more advanced method, differential privacy adds random noise to datasets, making it mathematically improbable to identify any specific individual within a dataset. This approach allows AI models to gain insights from large data sets without exposing details about individual data points. Differential privacy is especially useful when organizations want to analyze trends and patterns in user behavior without compromising user anonymity. For instance, tech companies like Apple have used differential privacy to collect usage data for product improvement while preserving user privacy.
Explainability and Transparency
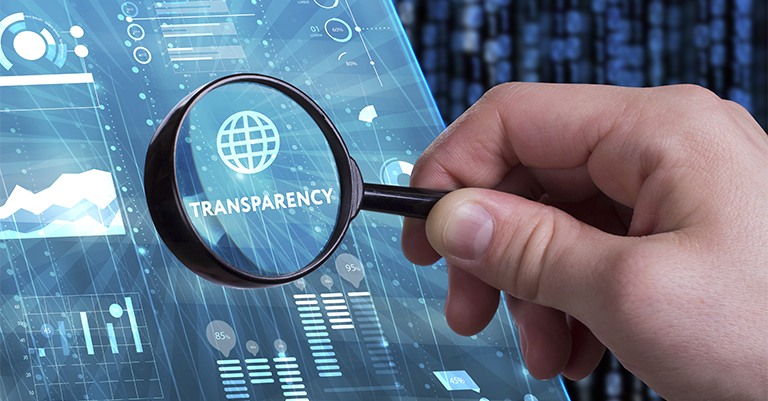
One of the significant challenges in AI is the “black box” nature of many advanced models, especially deep learning systems, where the decision-making process is not easily interpretable. Improving transparency and explainability can help users understand how their data is being used and how AI systems reach their decisions, building trust in the process.
Explainable AI (XAI)
XAI focuses on developing models that can provide clear explanations of their decisions and predictions. For instance, an AI system used in a bank for credit scoring could explain why it approved or denied a loan, listing the factors that influenced its decision. This not only helps users understand the reasoning behind AI-driven outcomes but also allows for better auditing and accountability.
Transparency Reports
Companies can issue transparency reports that outline how they collect, store, and use data within their AI systems. These reports can include information about the types of data collected, the purposes for which the data is used, and the retention period. Transparency reports can also disclose how AI models are trained and validated to ensure fairness and accuracy. By making this information publicly available, organizations can foster a sense of openness and accountability.
User Education and Consent Management
A key aspect of transparency is ensuring that users are fully aware of how their data is being collected and used. Consent management tools should be designed to make it easy for users to opt-in or opt-out of data collection practices. This involves providing users with clear, non-technical explanations of what they are consenting to, allowing them to make informed decisions about their privacy.
User-Centric Approaches
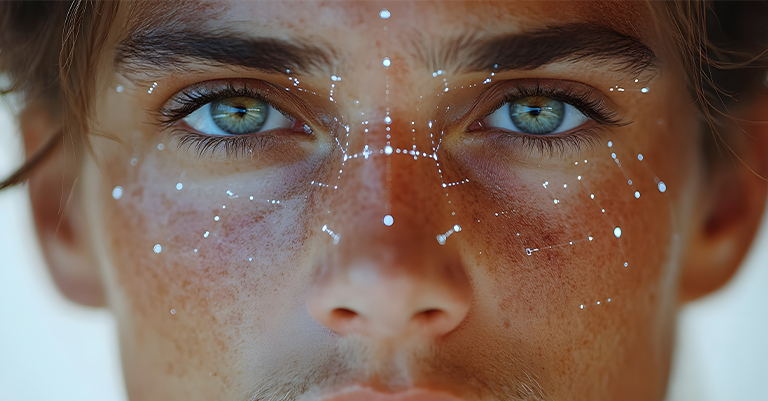
To ensure privacy, it’s essential to empower users with control over their own data. User-centric approaches involve giving individuals more authority over their personal information, making them active participants in managing their privacy.
Personal Data Management Tools
These tools enable users to access, view, and manage the data that has been collected about them. For instance, platforms could provide dashboards where users can see their activity history, delete data, or change privacy settings. This ensures users have a better grasp of what information is being stored and gives them the ability to adjust their preferences as they see fit.
Granular Consent
Offering granular consent allows users to choose which types of data they want to share and which they do not. For example, an AI-driven fitness app might allow users to share their exercise data but not their location history. This approach respects user autonomy and ensures that users share only the data they are comfortable with, aligning with the principles of informed consent.
Empowering Data Portability
Data portability enables users to transfer their data between services in a structured and usable format. This can be particularly valuable if users wish to switch to a service that they feel offers better privacy protections. Data portability promotes healthy competition among service providers and encourages businesses to maintain high standards of data protection to retain users.
Federated Learning
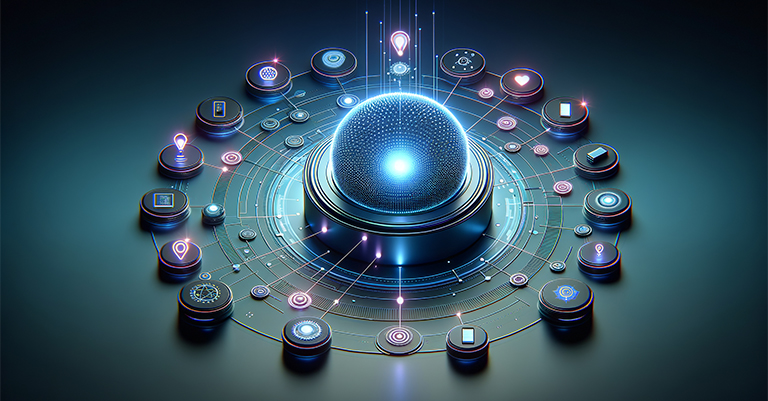
Federated learning is an innovative approach that allows AI models to be trained across multiple decentralized devices without the need to centralize the data. This technique significantly reduces the risks associated with central data storage and can be particularly beneficial for privacy-sensitive applications.
How Federated Learning Works
Instead of sending raw data to a central server for model training, federated learning sends the model to users’ devices, where it learns directly from the data stored locally. The updated model parameters are then sent back to a central server, where they are aggregated with updates from other devices. This ensures that the raw data never leaves the user’s device, reducing the risk of data exposure.
Privacy Benefits
Federated learning is particularly useful in scenarios where data is highly sensitive, such as in healthcare or personalized recommendations. For example, a health app might use federated learning to improve its diagnostic algorithms based on user data without ever directly accessing the data itself. This technique can help companies comply with data privacy regulations by minimizing the data that needs to be stored centrally.
Challenges and Implementation
While federated learning offers significant privacy advantages, it also presents challenges in terms of communication efficiency and computational power. Training models on user devices requires ensuring that devices can handle the computational load without draining resources. Despite these challenges, federated learning is gaining popularity as a solution for privacy-conscious AI development.
Ethical AI Training Programs
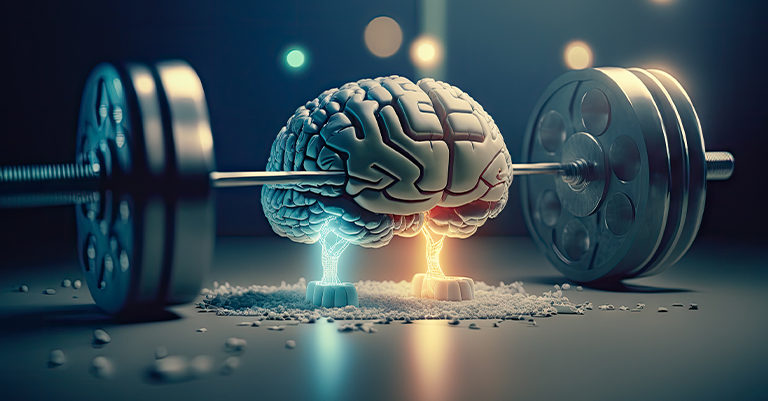
Ensuring that AI systems respect privacy goes beyond technical measures; it also requires fostering an organizational culture that values ethical considerations. Training AI developers and conducting regular audits can help align AI practices with ethical standards and privacy laws.
Ethics Training for AI Developers
Training programs focused on ethics can help developers understand the implications of privacy in AI and the importance of designing systems that respect user rights. These programs can cover topics like fairness, bias detection, and privacy-enhancing technologies, equipping developers with the knowledge they need to create more responsible AI systems. For example, developers can learn how to build models that avoid discriminatory biases, ensuring that AI decisions do not disproportionately affect certain groups.
Internal Audits and Compliance Checks
Regular internal audits can help organizations ensure that their AI systems comply with privacy regulations and ethical guidelines. Audits can evaluate how data is collected, stored, and processed, identifying potential privacy risks before they become issues. Compliance checks can also involve reviewing AI algorithms for fairness, ensuring that outcomes are not biased or discriminatory. This practice is particularly important in sectors like finance, healthcare, and recruitment, where AI decisions can have significant impacts on individuals.
Establishing AI Ethics Committees
Some organizations are setting up AI ethics committees to oversee the deployment of AI systems. These committees, which often include experts in law, technology, and ethics, provide guidance on the responsible use of AI, helping to navigate the complex ethical dilemmas that arise. For example, an ethics committee might review the potential privacy implications of deploying a new AI-powered surveillance system in public spaces.
Future of AI Privacy: Challenges and Opportunities
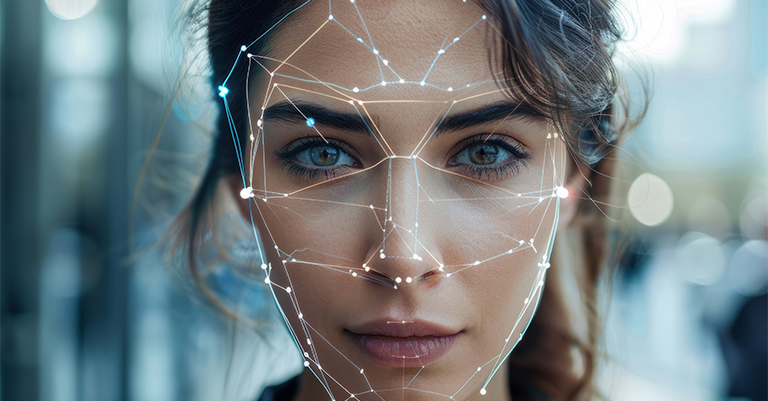
As artificial intelligence (AI) evolves, balancing its benefits with user privacy remains a key issue. The future of AI and privacy offers both challenges and opportunities, needing innovative solutions and collaboration to ensure privacy standards match technological progress. Below, we explore some of the most urgent challenges and promising opportunities in AI privacy.
Challenges
Advances in AI Capabilities
As AI systems become more advanced, their ability to make decisions without human input adds new challenges to data privacy. Applications like self-driving cars, intelligent healthcare diagnostics, and AI-driven financial services need large amounts of personal data to operate effectively. This autonomy in decision-making can create a gap between users and the systems managing their data.
Complex Decision-Making
AI systems using complex models like deep learning can analyze large datasets to generate insights and predictions. However, their decision-making process often lacks transparency. These “black box” models make it hard for users to understand how their data is processed, creating challenges in meeting data privacy regulations.
AI in Sensitive Sectors
In fields like healthcare, finance, and law enforcement, AI decisions can greatly impact individuals’ lives, making data privacy crucial. For example, AI in predictive policing may analyze large datasets, like historical arrest records, to predict potential criminal activity, but this raises concerns about surveillance and data misuse.
AI’s growing capabilities require new strategies for oversight, transparency, and accountability. This ensures that privacy rights are respected as AI makes more complex decisions.
Global Variation in Regulations
The varying approaches to data privacy regulations across different countries create a challenging landscape for companies that operate globally. While some regions, like the European Union, have stringent data protection laws such as the General Data Protection Regulation (GDPR), other countries may have less comprehensive or differing standards.
Compliance Complexity
Multinational companies must navigate a complex set of regulations, adjusting their data handling practices to each region’s requirements. This can create significant administrative burdens and increase legal risks, as non-compliance in one area could lead to heavy fines and penalties.
Uneven Protection Standards
The disparity in privacy standards means that users in different parts of the world may receive varying levels of data protection. For example, users in the EU benefit from robust rights under the GDPR, including the right to access, rectify, and erase their data. In contrast, users in regions with less stringent regulations may have fewer protections, leading to an imbalance in global privacy standards.
Cross-Border Data Transfers
AI systems often use cloud infrastructure that spans multiple countries, making secure data transfer across borders a major challenge. Differing regulations on data transfer, like the EU’s restrictions on sending personal data to non-compliant countries, require companies to implement extra safeguards. This adds complexity to global data management.
These varying regulations show the need for a more unified approach to privacy laws. A harmonized system could offer consistent user protection worldwide while simplifying compliance for companies.
Emerging Technologies
New technological advancements, such as quantum computing and 5G networks, present both opportunities and threats to the security and privacy of data in AI systems.
Quantum Computing
Quantum computing could revolutionize data processing, solving complex problems that classical computers can’t handle today. However, it also threatens existing encryption methods. Many encryption standards that protect data now could become ineffective once quantum computers reach a certain capability. This shift makes developing quantum-resistant cryptographic techniques crucial.
5G and IoT Expansion
The rollout of 5G networks and the proliferation of Internet of Things (IoT) devices increase the volume and velocity of data being generated. While this enables AI to analyze data in real-time, it also expands the potential attack surface for cybercriminals. As more devices collect and transmit personal data, ensuring secure communication and data storage becomes increasingly challenging.
AI-Generated Synthetic Data
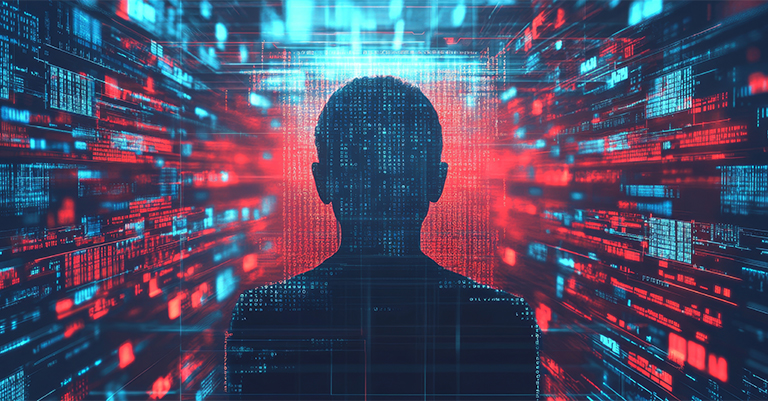
AI itself can generate synthetic data, which is often used to train models when real data is limited or sensitive. While synthetic data can help address privacy concerns by providing anonymized datasets, there is still the risk that poorly generated synthetic data could inadvertently reveal patterns or information about individuals.
Addressing the risks posed by emerging technologies requires a proactive approach to security, including investment in new cryptographic methods and an emphasis on secure design principles for AI systems.
Opportunities
Collaboration Between Governments and Tech Firms
The complexity of AI privacy issues makes it hard for any single entity—whether a government or a private company—to address them alone. Collaboration between governments, tech firms, and academic institutions can help create standards and regulations that are practical and effective.
Unified Privacy Standards
Collaboration can establish international data privacy standards that go beyond regional differences, making compliance easier for global companies and ensuring consistent data protection for users worldwide. For example, organizations like the International Organization for Standardization (ISO) develop standards that can be adopted globally.
Public-Private Partnerships
Public-private partnerships can foster innovation in privacy-preserving technologies, like new encryption methods, homomorphic encryption, or AI systems that follow current privacy laws. Governments can offer regulatory frameworks, while tech firms bring their AI expertise.
Regulatory Sandboxes
Some governments have introduced “regulatory sandboxes,” allowing companies to test new technologies in a controlled environment with regulator supervision. This approach is especially useful for developing AI applications that handle sensitive data, helping innovators ensure compliance before broader use.
These collaborations can create adaptive regulations that keep pace with the fast-changing AI landscape. They also ensure privacy protections are built into AI systems from the start.
AI in Privacy Protection
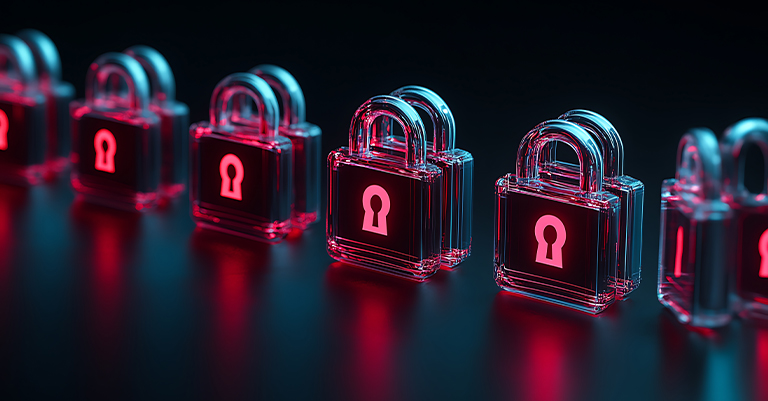
While AI is often seen as a threat to privacy, it can also be used as a tool to enhance data security and safeguard privacy. The use of AI for monitoring and detecting privacy threats can lead to more robust protection mechanisms.
Anomaly Detection
AI can monitor data access patterns and detect anomalies that may indicate security breaches or unauthorized access. For example, AI-based intrusion detection systems can spot unusual network traffic behaviors that might indicate a hacking attempt, allowing for quick responses to potential threats.
Automated Compliance Tools
AI can help companies comply with privacy regulations by automating data management tasks, such as tracking user consent, managing data access requests, and processing data deletion requests quickly. This approach simplifies compliance and reduces the risk of human error.
Privacy-Preserving Computation
Techniques like federated learning and secure multi-party computation use AI to analyze data while keeping it encrypted and decentralized. These methods let organizations gain insights from data without accessing the actual information, balancing the need for data analysis with privacy demands.
Leveraging AI for privacy protection represents a shift towards using the technology to solve some of the very challenges it creates, turning a potential risk into a valuable tool for safeguarding user data.
Consumer Awareness and Demand
As users become more aware of their digital footprint and privacy rights, they are increasingly demanding greater transparency and control over how their data is used. This shift in consumer expectations presents opportunities for companies to differentiate themselves through privacy-conscious practices.
User Empowerment
Growing awareness of data privacy can encourage users to make informed choices about the services they use and how their data is handled. For companies, being transparent about data practices is not just about compliance; it’s also a way to build user trust and loyalty.
Market Differentiation
Businesses that prioritize privacy and offer enhanced control over personal data can use this as a unique selling point. For example, some tech companies have begun marketing their products with a focus on privacy, such as offering encrypted messaging services or secure data storage solutions. This can attract users who value privacy, providing a competitive edge.
Influence on Regulatory Trends
As consumers advocate for greater privacy protections, they can influence regulatory trends, pushing for stronger data privacy laws and enforcement. This increased pressure can lead to more robust regulatory frameworks, which can benefit the entire ecosystem by providing clearer guidelines for responsible data use.
This heightened consumer demand for privacy aligns with a broader societal shift towards data ethics, emphasizing the importance of handling user information responsibly.
Navigating the Future: A Balanced Approach
The future of AI privacy requires balancing innovation with respect for user rights. Addressing the challenges and seizing opportunities needs a diverse approach that includes technical solutions, regulatory foresight, and active consumer engagement. This way, stakeholders can ensure that AI continues to develop while respecting core privacy and security principles. With thoughtful collaboration and a commitment to ethical practices, the AI community can build a digital world where technology benefits humanity without sacrificing individual freedoms.
Closing Thoughts: Balancing Innovation with Privacy
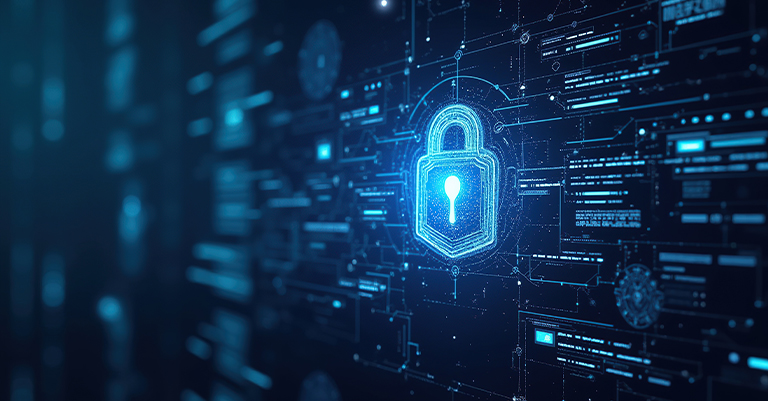
The rise of AI has brought many benefits, transforming industries like healthcare, finance, and education. However, these advancements also bring challenges, especially regarding privacy. AI systems can process large amounts of data and analyze behavior with great accuracy. This drives innovation but poses risks like data misuse, surveillance, and reduced individual autonomy. As these systems become more advanced, protecting privacy becomes even more critical.
Balancing AI’s power with privacy requires multiple strategies. Governments must create regulations that keep up with technological changes, ensuring privacy laws remain effective and relevant. Organizations and developers should adopt privacy-focused practices, such as anonymizing data and using privacy-by-design frameworks. They must also be transparent about how they collect and use data. Users must understand their rights and advocate for better privacy protections.
Moving forward requires collaboration between governments, organizations, developers, and users. This ensures AI respects privacy while still driving innovation. By adopting smart strategies and maintaining ethical standards, we can create a future where AI’s potential is fully realized without sacrificing privacy. This approach can build user trust and ensure AI’s benefits reach everyone, fostering a safer and more equitable technological landscape.